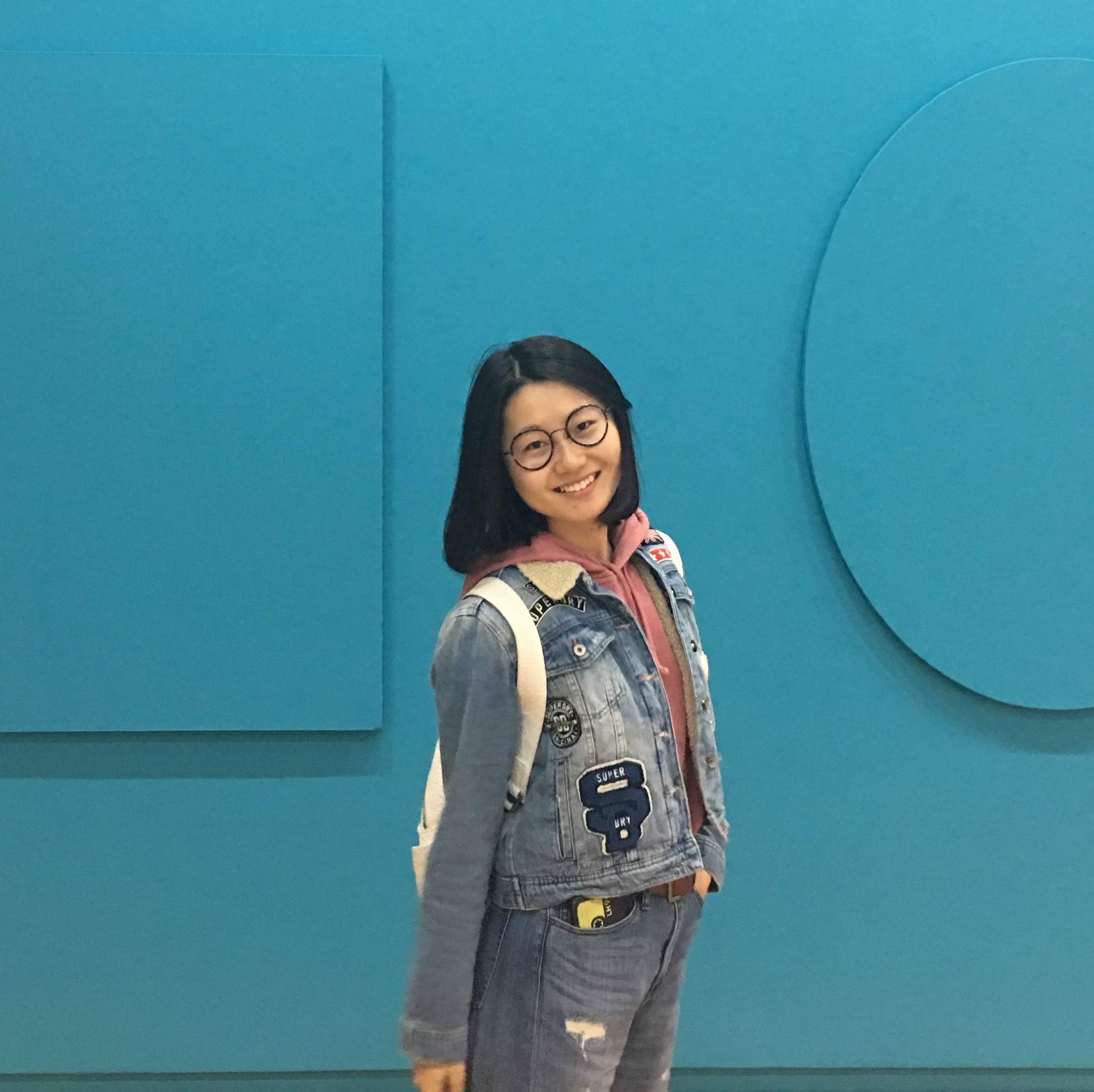
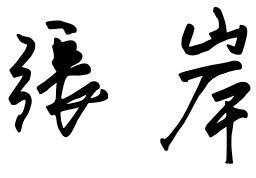
Professor
Alpha Lab
School of Information Science and Technology
University of Sicence and Technology of China (USTC)
Email: an.zhang3.14 at gmail.com
• Google Scholar Page • OpenReview Profile
Biography
I am currently a Professor at University of Science and Technology of China (USTC), where I lead the Alpha Lab. Prior to that, I was a postdoctoral research fellow in NExT++ Lab, National University of Singapore, working with Prof. Tat-Seng Chua. I obtained my Ph.D. from the National University of Singapore in 2021 and joined USTC in 2025. With my colleagues, students, and collaborators, we strive to develop foundation models (large language models & diffusion models) and AI Agents, with an emphasis on understanding the capabilities and properties of next-generation general AI models. - Capabilities: complex reasoning, self-reflection, self-improving, scaling law, and multi-modal integration. - Key properties: interpretability, trustworthiness, efficiency. Our research is motivated by, and contributes to, applications in information retrieval (e.g., recommender system), education (e.g., smart campus), and security (e.g., AI Safety). I have over 30 publications appeared in top-tier conferences (e.g., NeurIPS, ICLR, WWW, KDD, ICML, SIGIR) and journals (e.g., TPAMI, TOIS). Moreover, I have served as the PC member for top-tier conferences including NeurIPS, SIGIR, ICLR, KDD, EMNLP, WWW, AAAI, ICML, and WSDM.
Prospective Intern Students
I am looking for highly motivated interns (visiting Ph.D., master, and undergraduate students) to work together on the trustworthiness and robustness of graphs, especially pre-training, interpretability, generalization, and causality, and their applications in real-world scenarios. Please feel free to send me your CV and transcripts, if you have interest. We are also actively looking for opportunities in research, partnership and commercialization in exciting data science projects.
News and Highlights
Robust Collaborative Filtering to Popularity Distribution Shift.
ReLM: Leveraging Language Models for Enhanced Chemical Reaction Prediction.
Empowering Collaborative Filtering with Principled Adversarial Contrastive Loss.
Evaluating Post-hoc Explanations for Graph Neural Networks via Robustness Analysis.
Rethinking Tokenizer and Decoder in Masked Graph Modeling for Molecules.
Redundancy-aware Transformer for Video Question Answering.
Online Distillation-enhanced Multi-modal Transformer for Sequential Recommendation.
Discovering Dynamic Causal Space for DAG Structure Learning.
Invariant Collaborative Filtering to Popularity Distribution Shift.
Boosting Causal Discovery via Adaptive Sample Reweighting.
Cooperative Explanations of Graph Neural Networks.
Incorporating Bias-aware Margins into Contrastive Loss for Collaborative Filtering.
Let invariant Rationale Discovery inspire Graph Contrastive Learning.
CrossCBR: Cross-view Contrastive Learning for Bundle Recommendation.
Reinforced Causal Explainer for Graph Neural Networks.
Discovering invariant rationales for graph neural networks.
Professional Services
Honors and Awards
Background
Supervisor: Prof Tat-Seng Chua & Prof Zhenkai Liang
Supervisor: Prof Tat-Seng Chua
Supervisor: Prof Zehua Chen
Supervisor: Prof Jinde Cao & Prof Wenwu Yu